What is Dagger?
Before we dive into our first hands-on lesson, we should try to understand the role and place of Dagger in the modern development workflow:
- What exactly is Dagger?
- Is it a service, a CLI tool, or maybe both?
- Is it a complete replacement for GitHub Actions/Jenkins/CircleCI?
- Or is it merely a dev tool that augments existing CI/CD providers?
- Can Dagger be self-hosted? If yes, what is Dagger Cloud for then?
- What makes Dagger so special that you might want to switch to it?
One effective way to answer these questions is to draw parallels between Dagger and familiar tools and services we use daily in our projects.
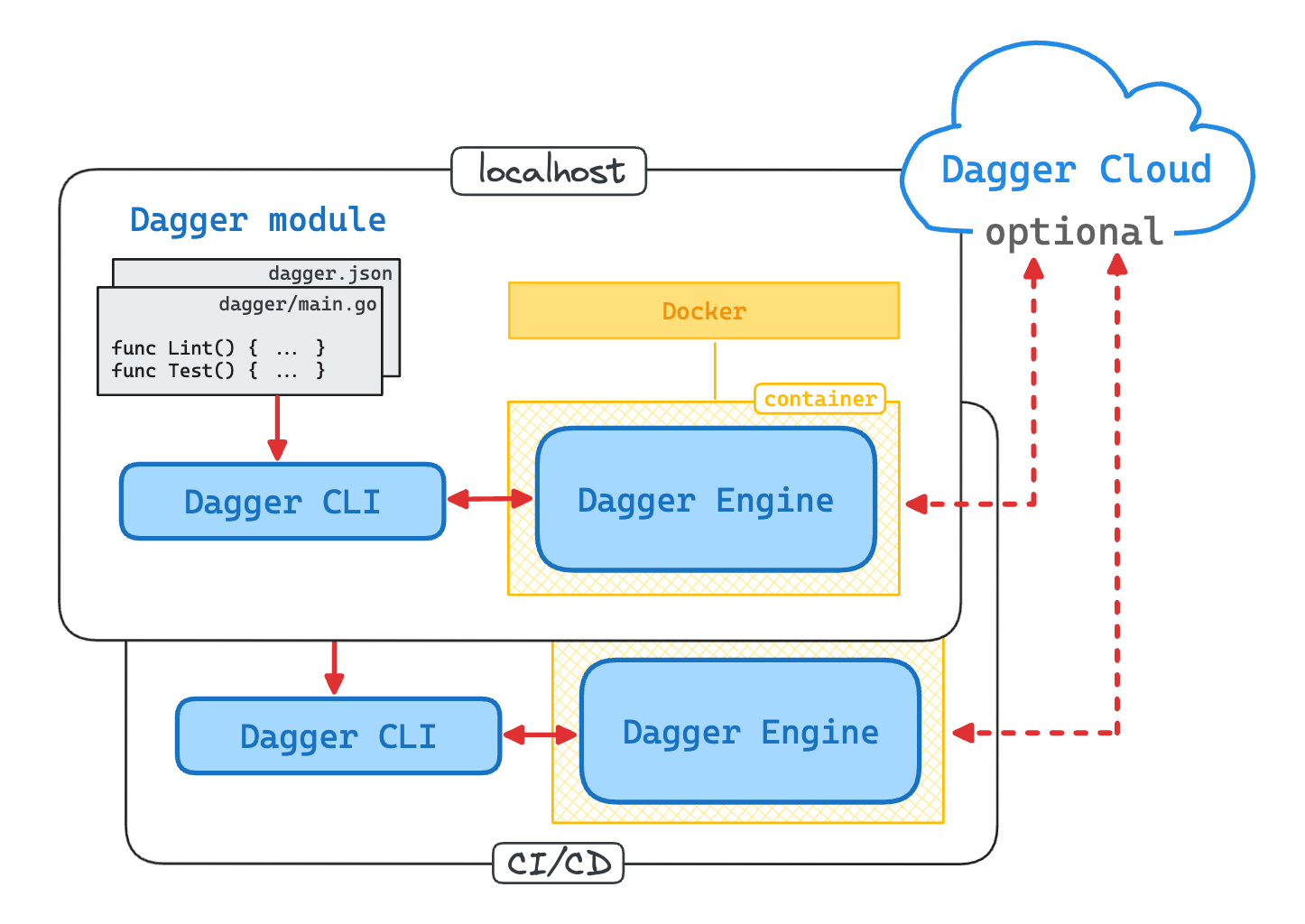
Framing the Problem
In almost any modern software development project, a few common tasks typically arise:
- Linting the code
- Running the tests
- Building the project
- Publishing images
- Etc.
While these tasks are often associated with CI/CD, they are actually part of the broader development workflow. For instance, developers often want to lint the code and run unit tests locally before pushing their changes and triggering the first CI pipeline. Automation of these typical development tasks with Dagger will be the cross-cutting theme of this course.
To illustrate the problems and compare the solutions, we will use a simple yet realistic software project,
iximiuz/todolist,
a TODO-as-a-service web app written in Go and JavaScript.
Its source code is conveniently checked out in the playground as ~/todolist
-
take a quick look at it to get a sense of the project structure.
First, let's see how the above development tasks are codified in Todolist without Dagger.
Development workflow before Dagger
Like many other projects, Todolist uses Make to organize its development workflow.
Here is how the linting, testing, and building steps are defined in the project's Makefile
:
cat ~/todolist/Makefile
.PHONY: lint
lint:
golangci-lint run ./...
.PHONY: test
test:
go test -v ./...
.PHONY: build
build:
CGO_ENABLED=0 go build -o server
...
π‘ Despite being half a century old, Make remains extremely ubiquitous. It was originally designed as a build tool but nowadays it's often (ab)used as a general purpose task runner, with PHONY targets used to essentially define tasks.
With all workflow tasks aggregated in a single Makefile, developers can handily run them locally:
make lint
# or
make test
# or
make build
...while in CI/CD, the same make targets can be invoked by the remote build servers:
# .github/workflows/lint.yml
...
jobs:
lint:
runs-on: ubuntu-latest
steps:
- uses: actions/checkout@v4
- uses: actions/setup-go@v2
- run: make lint # <-- the same `make lint` as on the developer's machine
The above approach is quite common - probably because it's easy to get started with, and because it works pretty well for small(er) projects. But when the project grows, its Makefile tends to become rather unwieldy and hard to maintain.
On the use of alternative task runners and refactoring Make targets into scripts π€
Over time, the development workflow tasks tend to become more complex. When a Make target becomes too lengthy, it can be refactored into a separate shell script that is then invoked by the original target. Thus, the following project structure is also pretty common:
ls my-advanced-project/hack
lint.sh test.sh build.sh
cat my-advanced-project/Makefile
.PHONY: lint
lint:
./hack/lint.sh
.PHONY: test
test:
./hack/test.sh
.PHONY: build
build:
./hack/build.sh
...
Using more modern task runners like Just or Task instead of Make can help further reduce the complexity. They often come with nice extra features and are devoid of the most annoying Make's peculiarities. However, they do not substantially change the situation, as they are subject to the same design limitations as Make. In the end, such a task runner is just a local process that executes scripts (or ad hoc commands) directly on its host machine.
Let's see where Dagger can come into play and improve the development workflow organization.
Development workflow with Dagger
The problem of over-complicated development workflows can be tackled from different angles and with varying levels of disruption to the existing processes. For instance, Dagger tries to solve it by replacing traditional automation tools like Make, Just, or Task, and the messy shell scripts they usually rely on, while allowing you to stay with your current CI/CD provider (GitHub Actions, CircleCI, etc.).
Dagger itself is an automation tool that leverages a similar to Docker's architecture:
- Local Dagger CLI and Dagger Engine components (open source).
- Optional Dagger Cloud integrations (proprietary, SaaS).
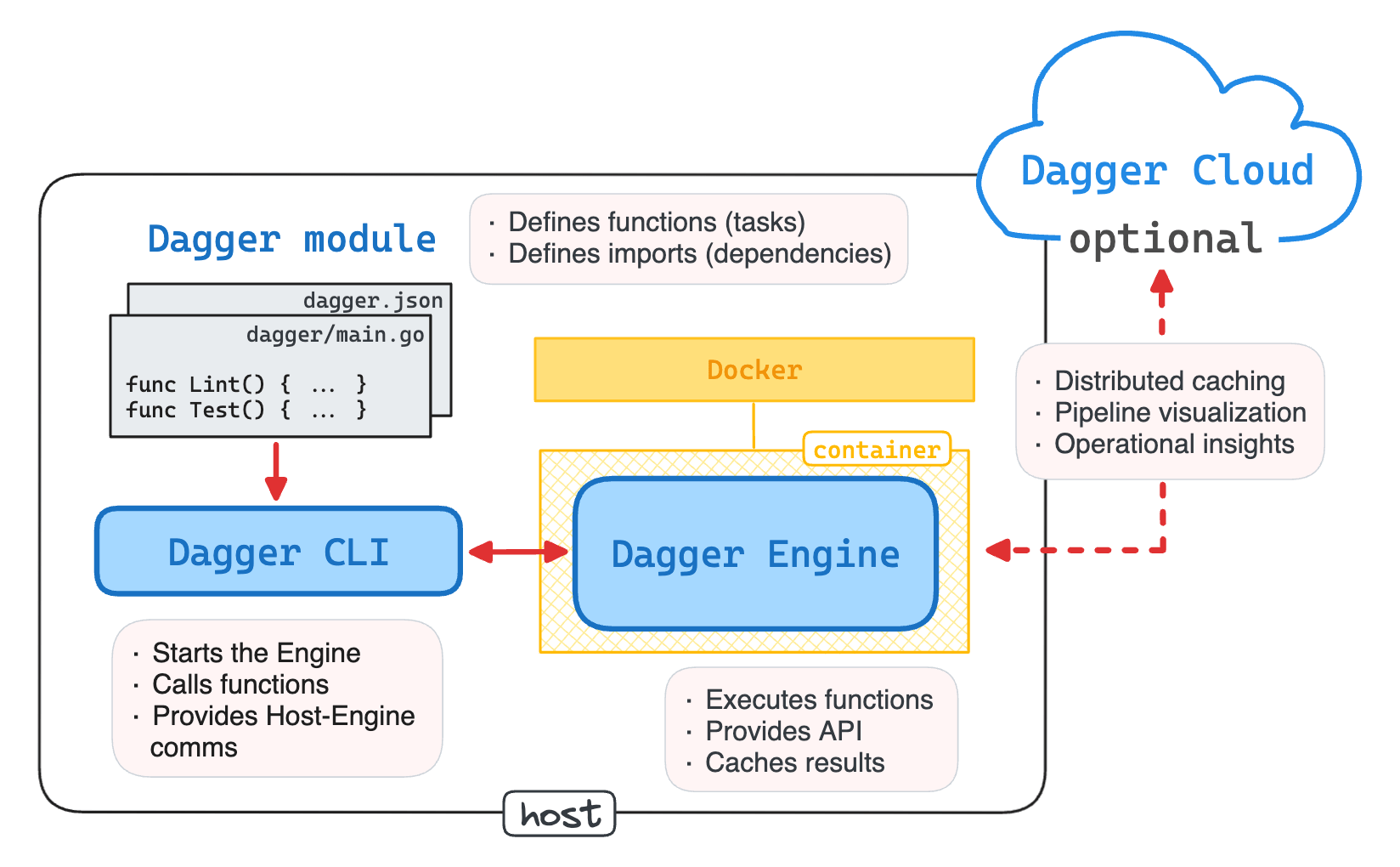
How to install Dagger CLI and Dagger Engine?
The only prerequisite for using Dagger is to download the Dagger CLI:
curl -L https://dl.dagger.io/dagger/install.sh | BIN_DIR=/usr/local/bin sh
The initialization of the Dagger Engine happens automatically when you run the Dagger CLI for the first time.
The engine is conveniently distributed as a container image, and the Dagger CLI relies on the local docker
CLI to pull and launch it.
Dagger can also work with other container runtimes like Podman or nerdctl - the only additional thing you need to do is to create an alias like below π
# For Podman
sudo ln -s $(which podman) /usr/local/bin/docker`
# For nerdctl
sudo ln -s $(which nerdctl) /usr/local/bin/docker`
Remote Dagger Engine execution is also supported, and we'll dig into the details of running Dagger Engine in Kubernetes in one of the future lessons.
Refer to the official documentation for the full list of supported runtimes and integrations.
Dagger's analog of Make targets (or Just's recipes, or Task's tasks, no pun intended), is Functions and Modules that can be written in a variety of supported languages (Go, Python, TypeScript, etc.)
Here is what Todolist's Dagger module might look like:
package main
type Todolist struct{}
func (m *Todolist) Lint() {
// Lint logic goes here...
}
func (m *Todolist) Test() {
// Test logic goes here...
}
func (m *Todolist) Build() {
// Build logic goes here...
}
Continuing drawing parallels with traditional task runners,
Dagger Functions can be invoked from the command line using the dagger call
command:
dagger call lint # formerly, make lint
# or
dagger call test # formerly, make test
# or
dagger call build # formerly, make build
...and the same Dagger Functions can also be executed on CI/CD servers:
# .github/workflows/lint.yml
...
jobs:
lint:
runs-on: ubuntu-latest
steps:
- uses: actions/checkout@v4
- uses: dagger/dagger-for-github@v5
verb: call # <-- same as `dagger call lint` on the developer's machine
args: lint
But so are Make targets, Just's recipes, and Task's tasks!
So, if Dagger is yet another task runner, perhaps with a little more expressive way of defining tasks, why anyone would want to use it instead of their current task runner of choice?
Why use Dagger?
The traditional "Make-based" workflow automation has a number of apparent and not-so-apparent drawbacks:
- Larger Makefiles and shell scripts are notoriously hard to read and maintain.
- Cross-task communication via environment variables is error-prone and insecure.
- Dirty local caches can spoil consecutive task executions.
- Leaking cross-project dependencies is hard to spot and debug.
- Tool versions drift across different dev machines and CI servers.
- Etc.
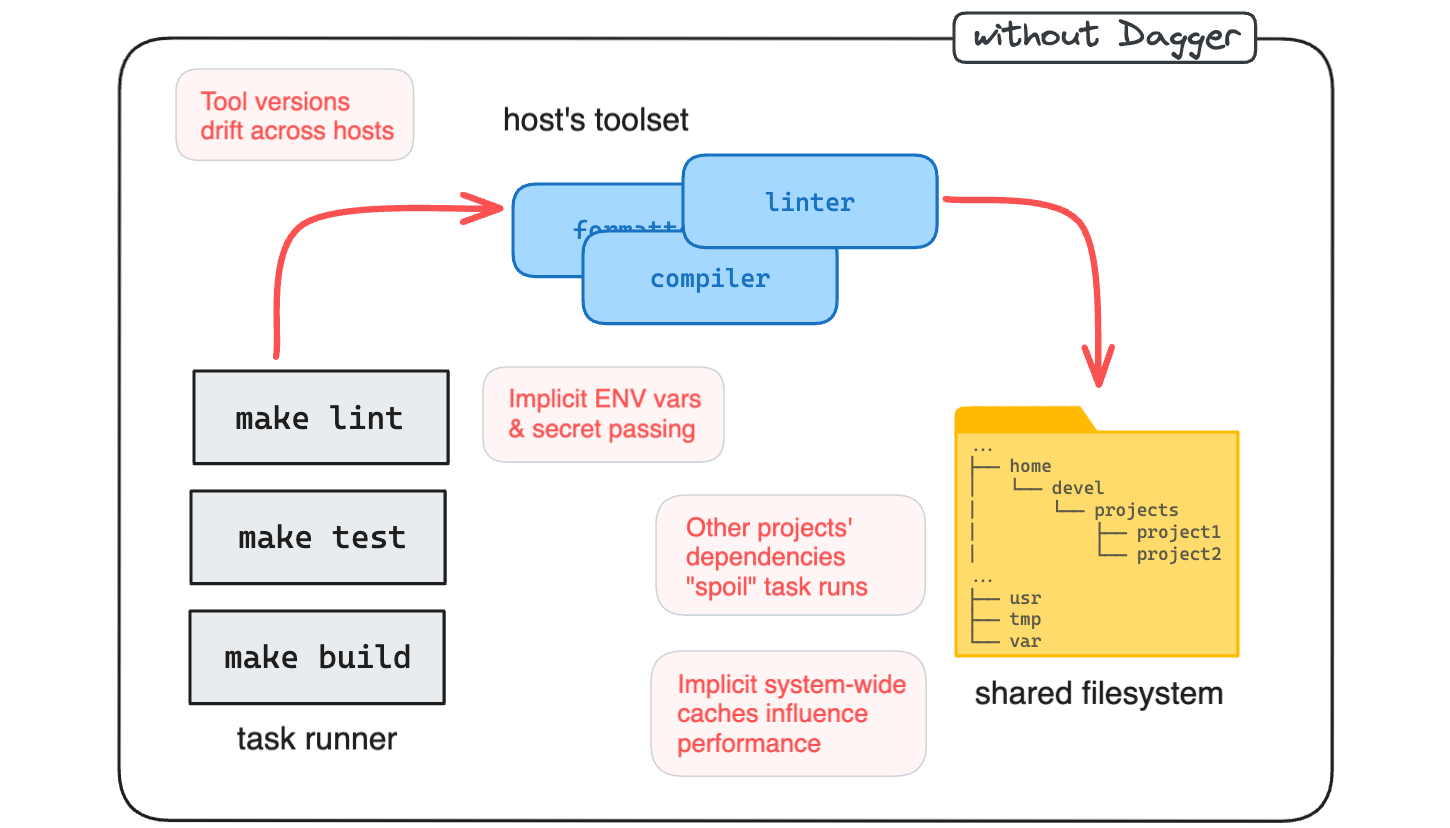
If we look closer at the above list, we can identify two major classes of issues with the traditional approach:
- (i) Readability and maintainability of the task definitions.
- (ii) Reproducibility of task executions across different machines.
Dagger aims to solve the first class of issues by using full-fledged programming languages like Go, Python, or TypeScript to write and interconnect tasks in a more developer-friendly way. However, this is not something only Dagger can do - Just supports writing its recipes in non-shell languages, too.
More interesting is how Dagger tries to solve the second class of issues. For that, it uses a rather unique approach - every task (i.e., a Dagger Function) is executed in its own container. This may sound a bit odd at first, but it's actually a very clever design decision.
While traditional task runners, potentially combined with scripts, ensure every engineer and CI server run exactly the same commands, Dagger goes one step further and ensures that these commands are executed in identical environments β€οΈβπ₯
Containerized execution of tasks solves one of the fundamental problems of development workflow automation: reproducibility of task executions across different machines. Dirty local caches, drifting tool versions, Linux vs. macOS discrepancies stop being an issue if you run your tasks with Dagger.
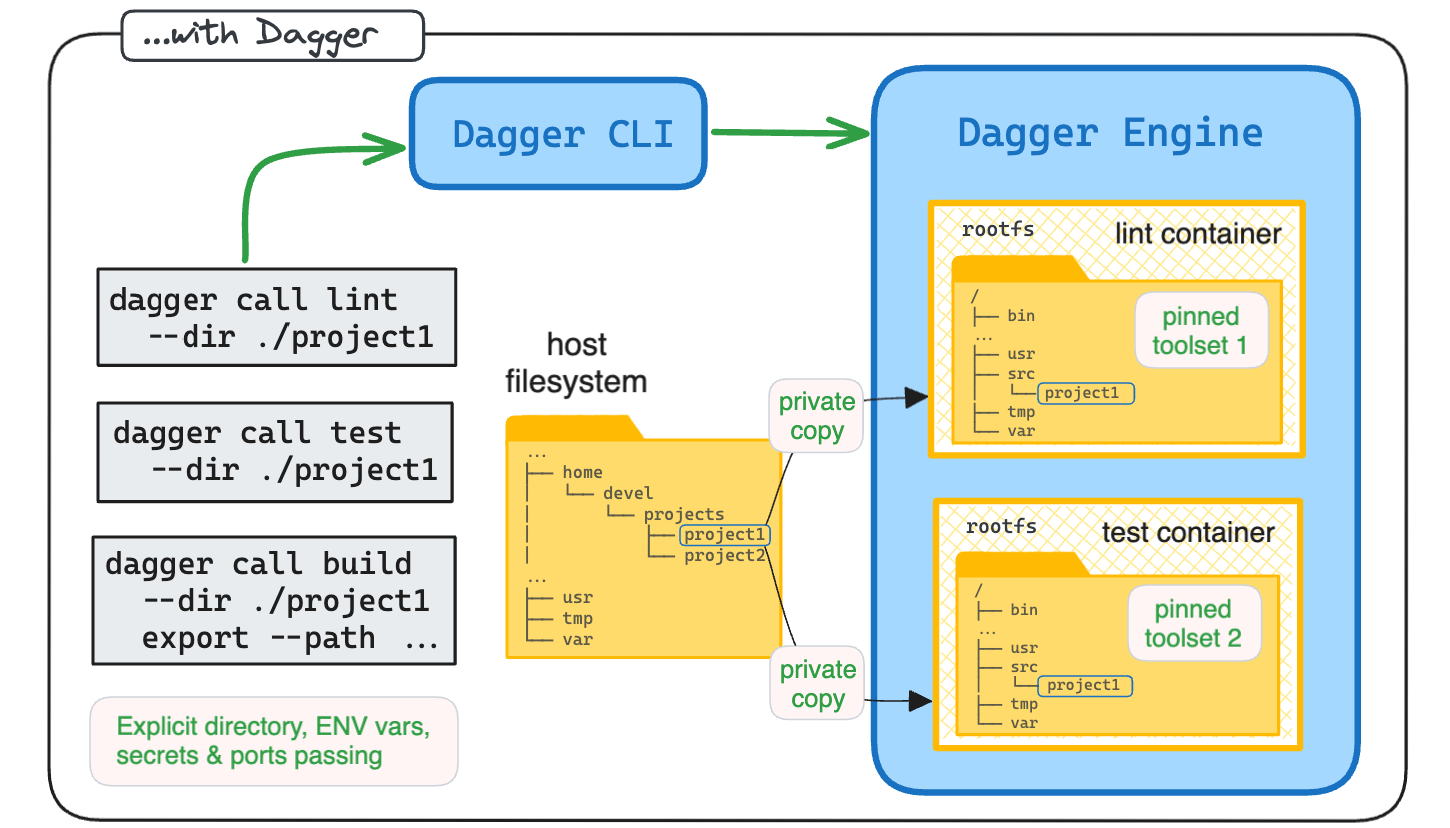
A nice byproduct of this design is that function call results can be efficiently cached. This makes the subsequent executions of the same function much faster when used locally, but it might be even more useful when used in ephemeral CI/CD environments, where (optional) integration with Dagger Cloud can seamlessly add a layer of distributed caching, eliminating the cold worker start issue.
π‘ By their nature, development workflow automation tasks usually modify the state of the local filesystem. When every task execution gets its own isolated filesystem (thanks to its container), the function call results can be easily cached by snapshotting the container's rootfs.
Another benefit of Dagger's containerized execution model is that Dagger can run tasks written in different languages as part of the same pipeline and even when the corresponding language runtimes aren't installed on the host machine. Additionally, because the function calling API is standardized, you can share your Dagger modules with other engineers and use modules written by other teams or the wider open-source community.
Lastly, since Dagger by design is "just a tool", it cannot replace CI/CD service providers like GitHub Actions, GitLab CI, Jenkins, or even Argo Workflows - someone still needs to supply compute resources and manage webhooks triggered by project events. But because Dagger is "just a tool", you can start using it locally and in existing CI/CD pipelines right away - no major upfront migration is required. And integration with Dagger Cloud can come in handy again bringing extra observability to your Dagger-powered pipelines.
Thus, there is plenty of really good reasons to give Dagger a try.
Summarizing
Dagger is a single-host automation engine that takes the place of traditional task runners like Make, Just, or Task and allows writing tasks in full-featured programming languages (Go, Python, TypeScript, etc.). It is conveniently distributed as a single statically linked binary (CLI), which, upon the first run, pulls and launches a container with the Dagger Engine (daemon). Dagger can be used on any host with Docker (or the like) runtime installed, locally and on remote CI workers. Additionally, Dagger can be augmented with Dagger Cloud - a hosted service that helps operate Dagger-powered CI/CD pipelines.
Dagger truly stands out due to its:
- Highly parallel, container-native, and portable execution model.
- The ability to write tasks (Dagger Functions) in real programming languages.
- The ability to import and reuse Dagger Functions written by others (see Daggerverse).
- Efficient on-disk local caching of function call results.
- Distributed caching and extra pipeline observability (optional, via Dagger Cloud).
Sounds like something you'd like to try? In the next lesson, we will write our first useful Dagger Function, learn how to call it from the CLI, and explore what happens from the user's perspective and under the hood.
But first, let's practice what we've learned so far!
Level up your Server Side game β Join 10,000 engineers who receive insightful learning materials straight to their inbox